Teaching Tailored to Talent: Adverse Weather Restoration via Prompt Pool and Depth-Anything Constraint
1The Hong Kong University of Science and Technology (Guangzhou) 2Xiamen University
3The Hong Kong University of Science and Technology
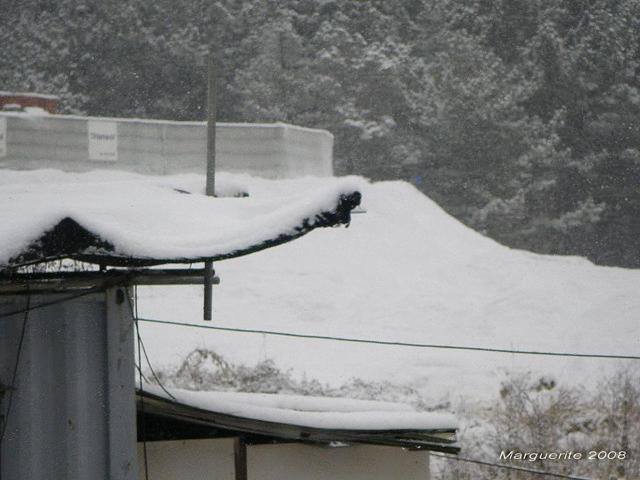
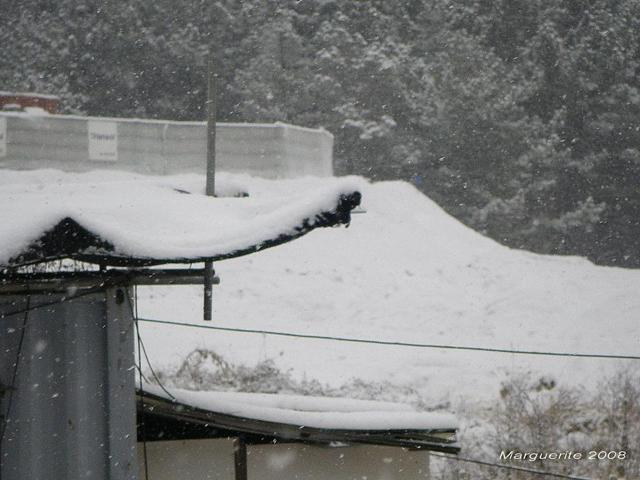
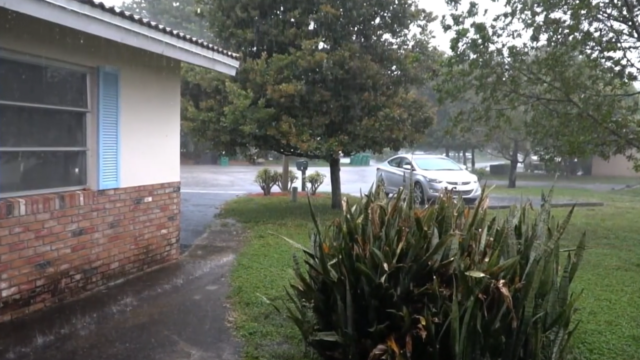
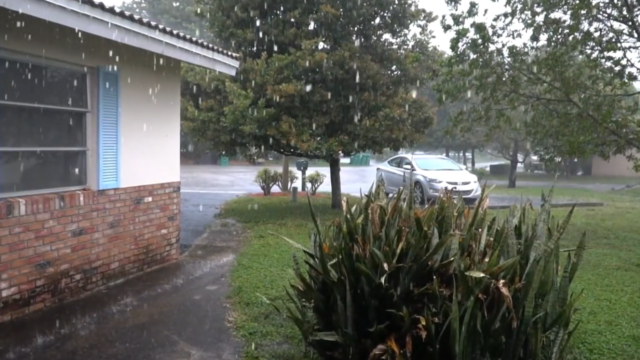
Abstract
Recent advancements in adverse weather restoration have shown potential, yet the unpredictable and varied combinations of weather degradations in the real world pose significant challenges. Previous methods typically struggle with dynamically handling intricate degradation combinations and carrying on background reconstruction precisely, leading to performance and generalization limitations. Drawing inspiration from prompt learning and the "Teaching Tailored to Talent" concept, we introduce a novel pipeline, T3-DiffWeather. Specifically, we employ a prompt pool that allows the network to autonomously combine sub-prompts to construct weather-prompts, harnessing the necessary attributes to adaptively tackle unforeseen weather input. Moreover, from a scene modeling perspective, we incorporate general prompts constrained by Depth-Anything feature to provide the scene-specific condition for the diffusion process. Furthermore, by incorporating contrastive prompt loss, we ensures distinctive representations for both types of prompts by a mutual pushing strategy. Experimental results demonstrate that our method achieves state-of-the-art performance across various synthetic and real-world datasets, markedly outperforming existing diffusion techniques in terms of computational efficiency.
Observations
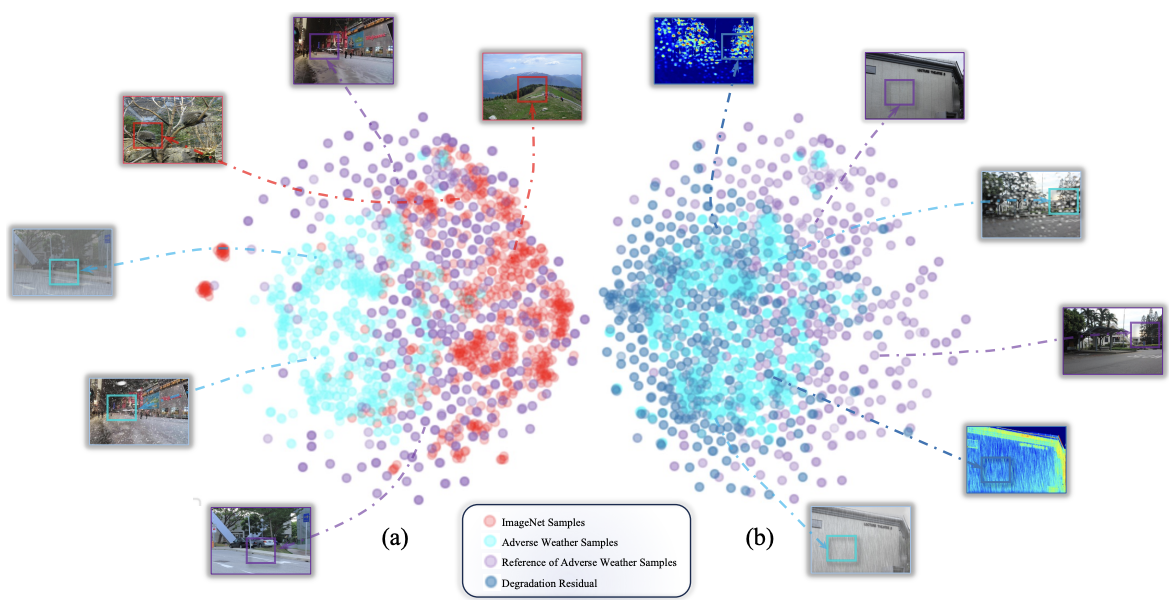
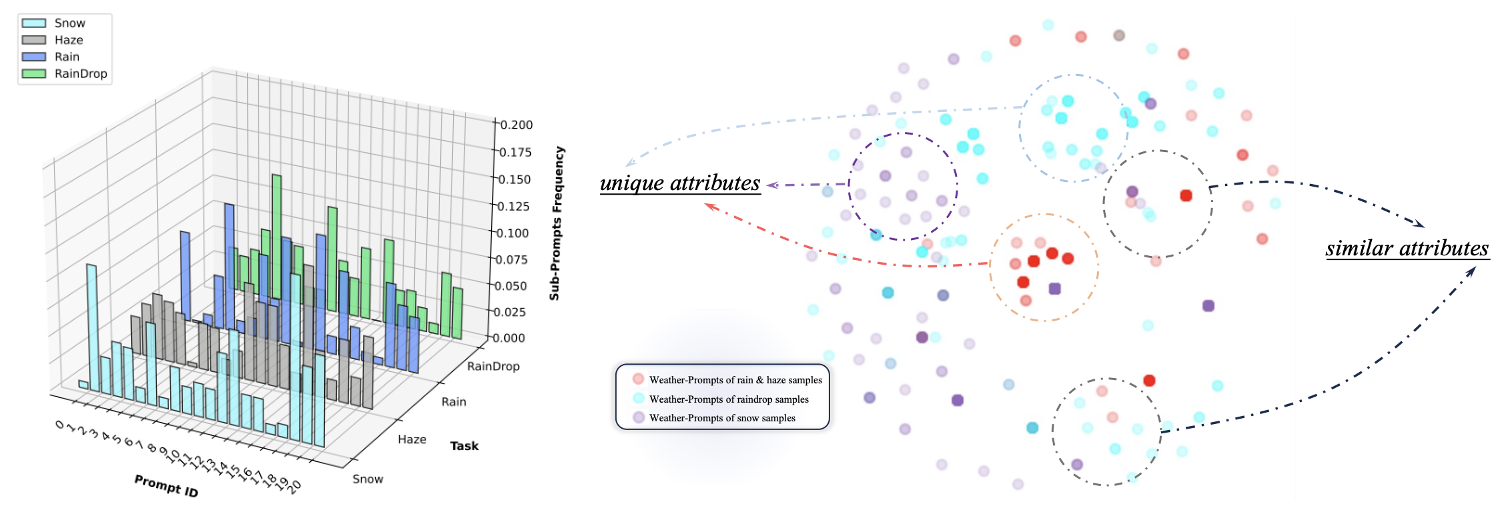
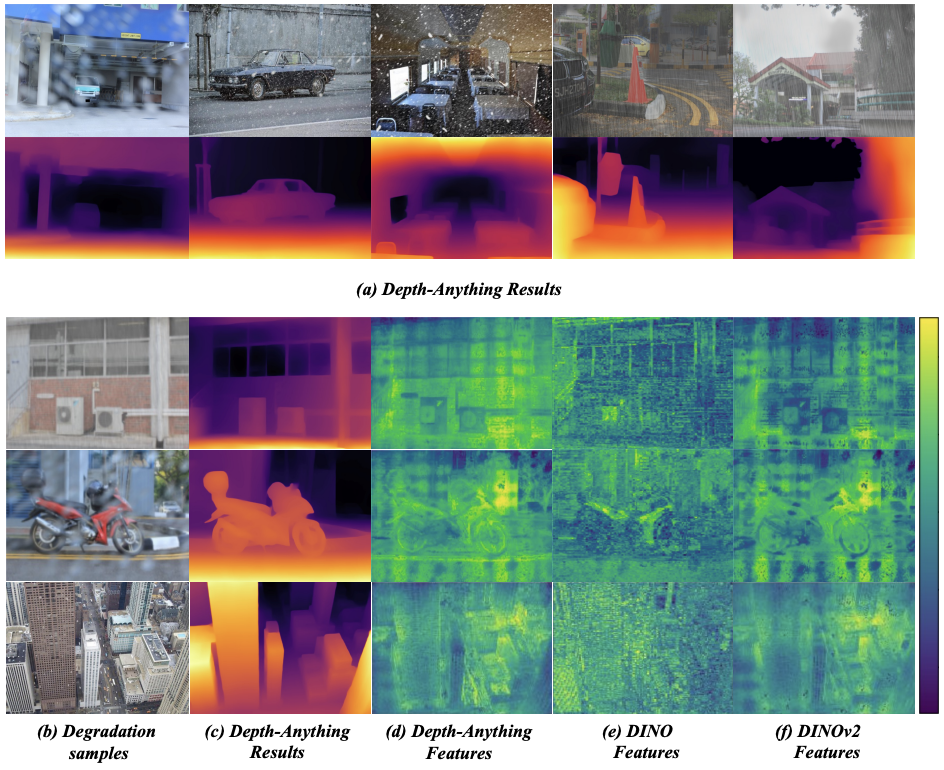
Mid Figure: t-SNE visualization of weather-prompts for different weather conditions.
Right Figure: Motivation of Depth-Anything as a constraint. Depth-Anything has degradation-independent performance, and the intermediate features have better robustness than the previous pre-trained network.
Method
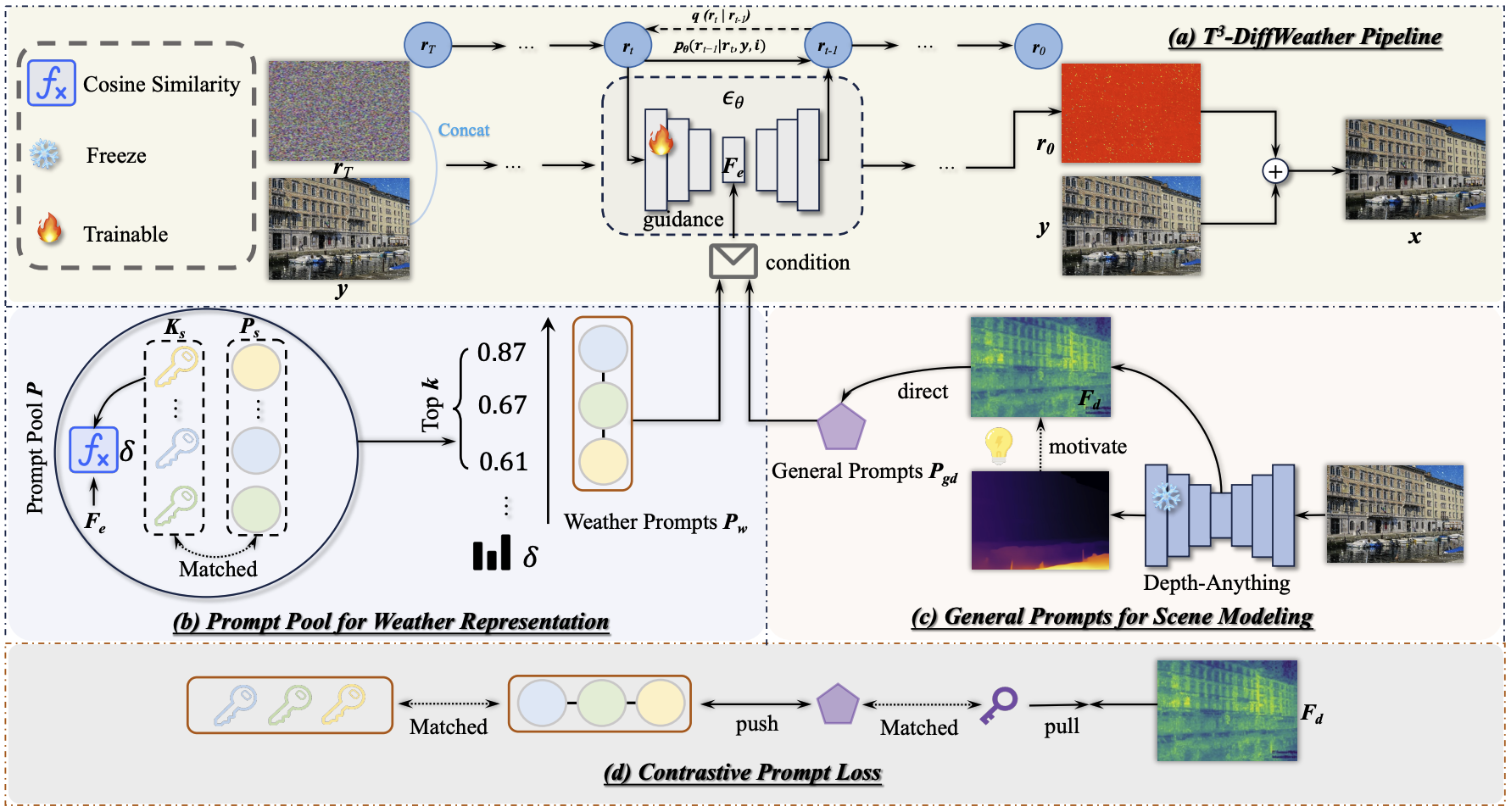
Quantitative Comparison
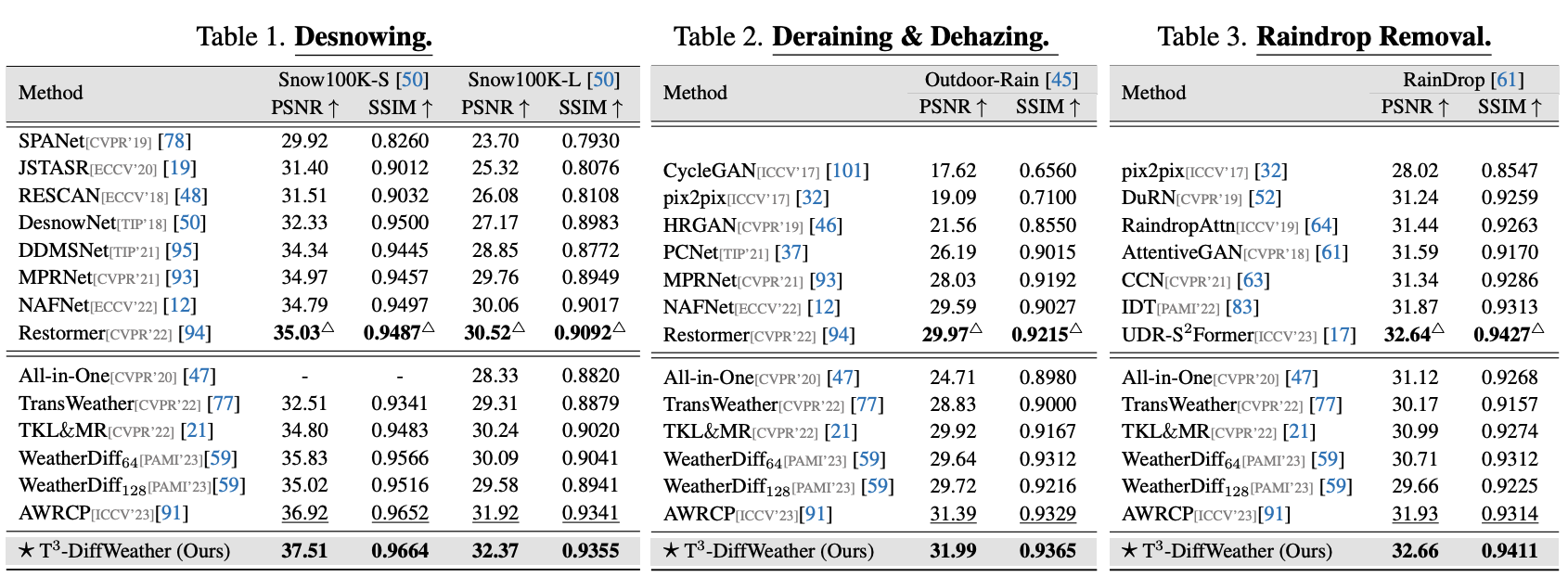
Visual Comparison
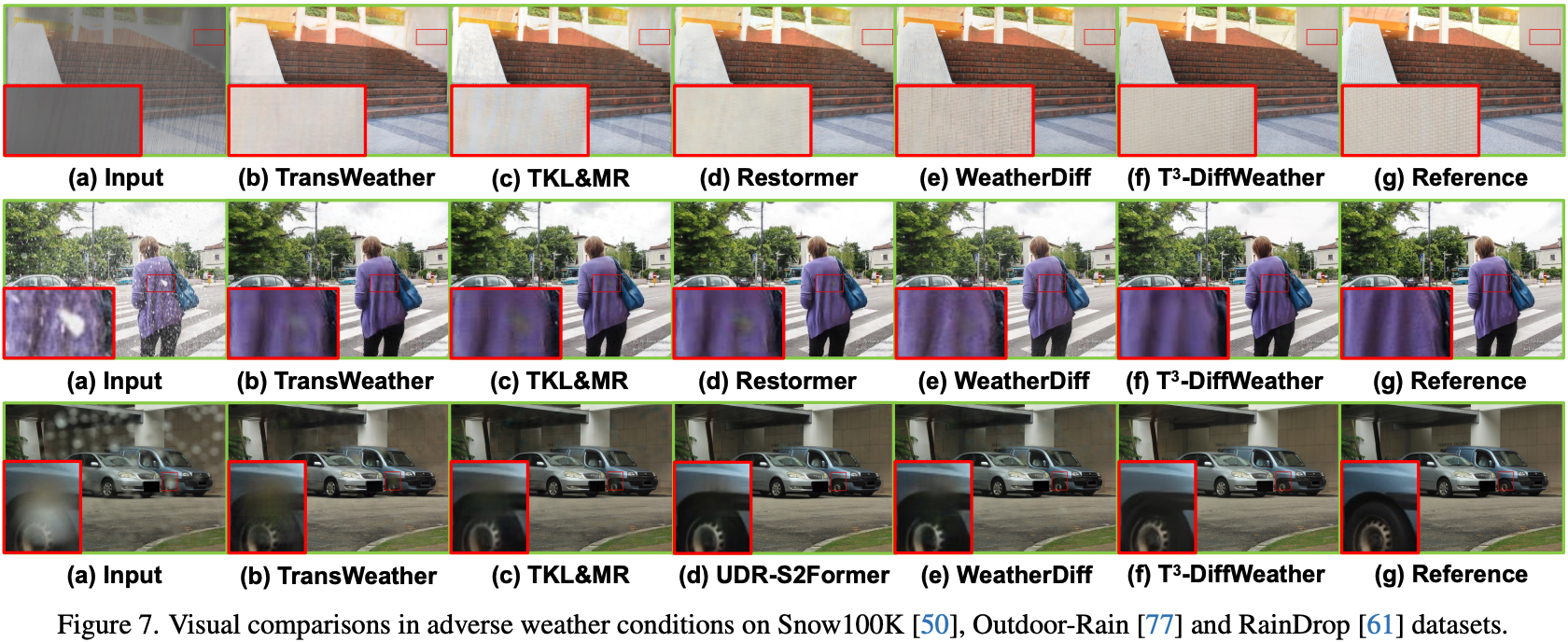
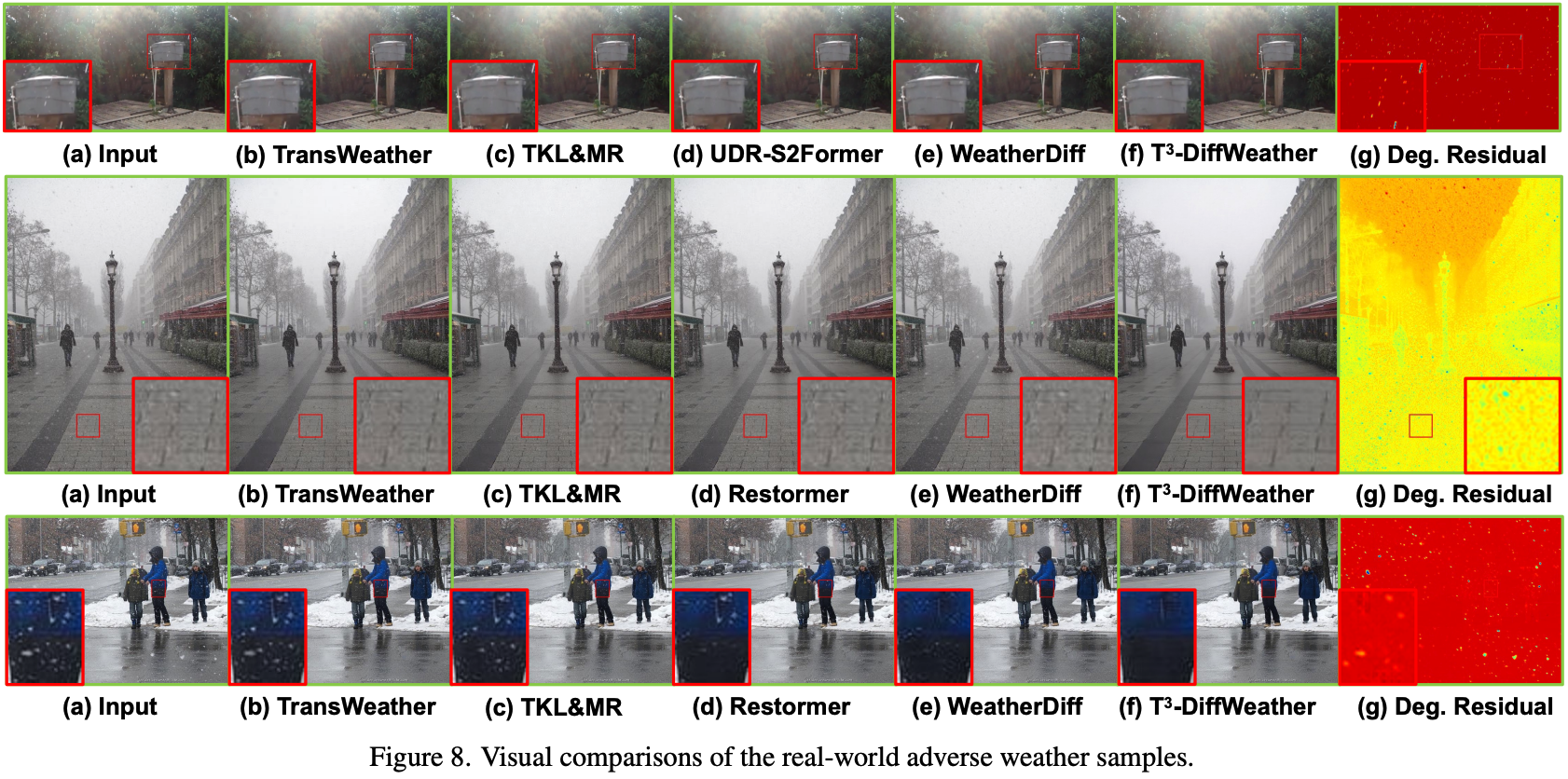
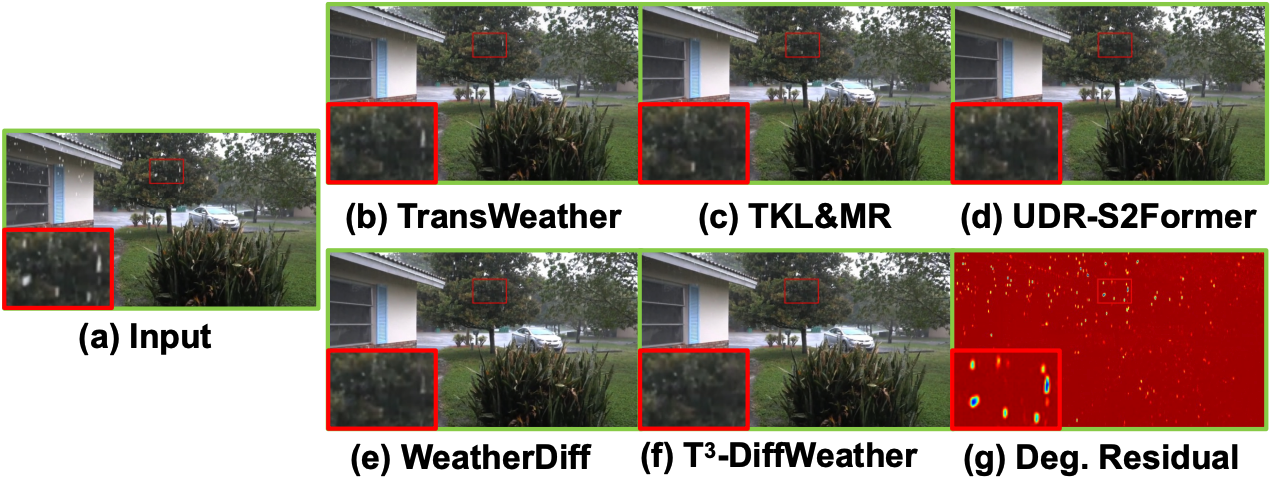
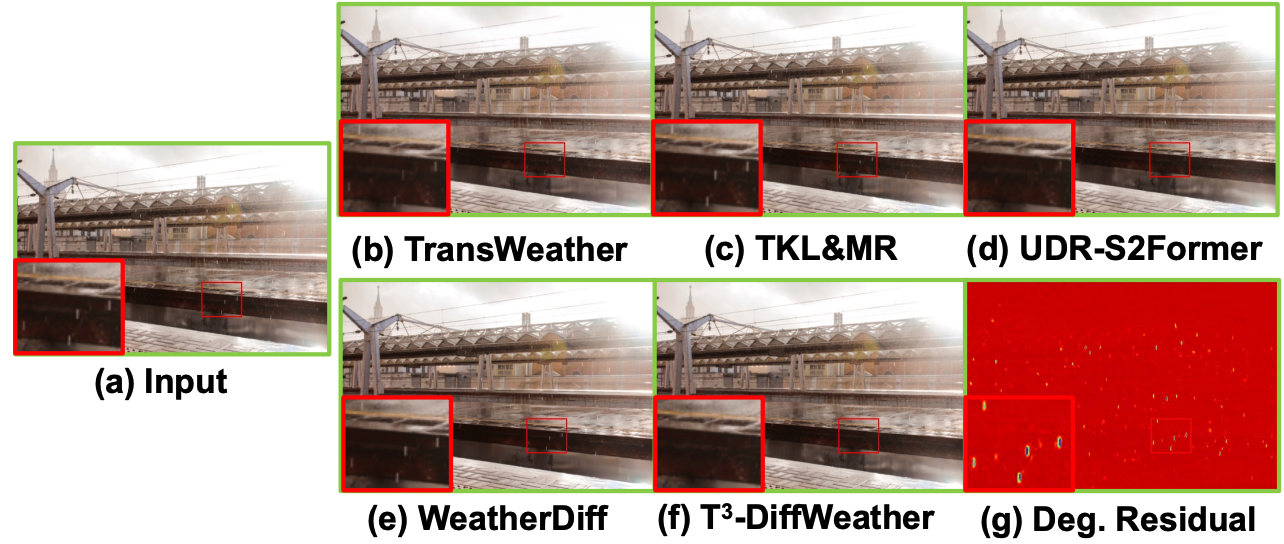
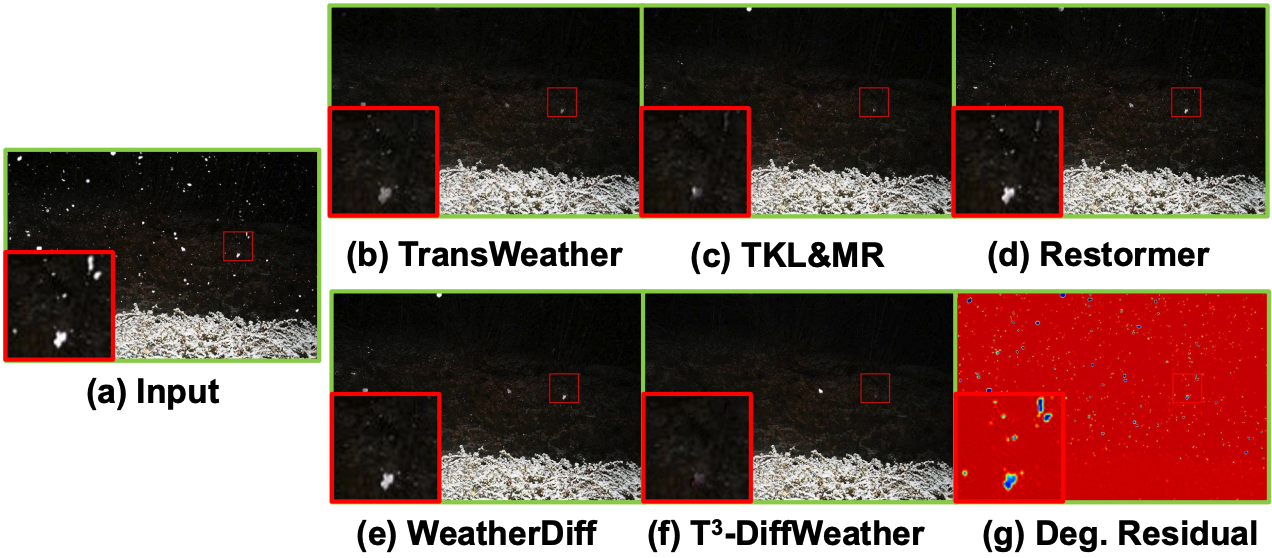
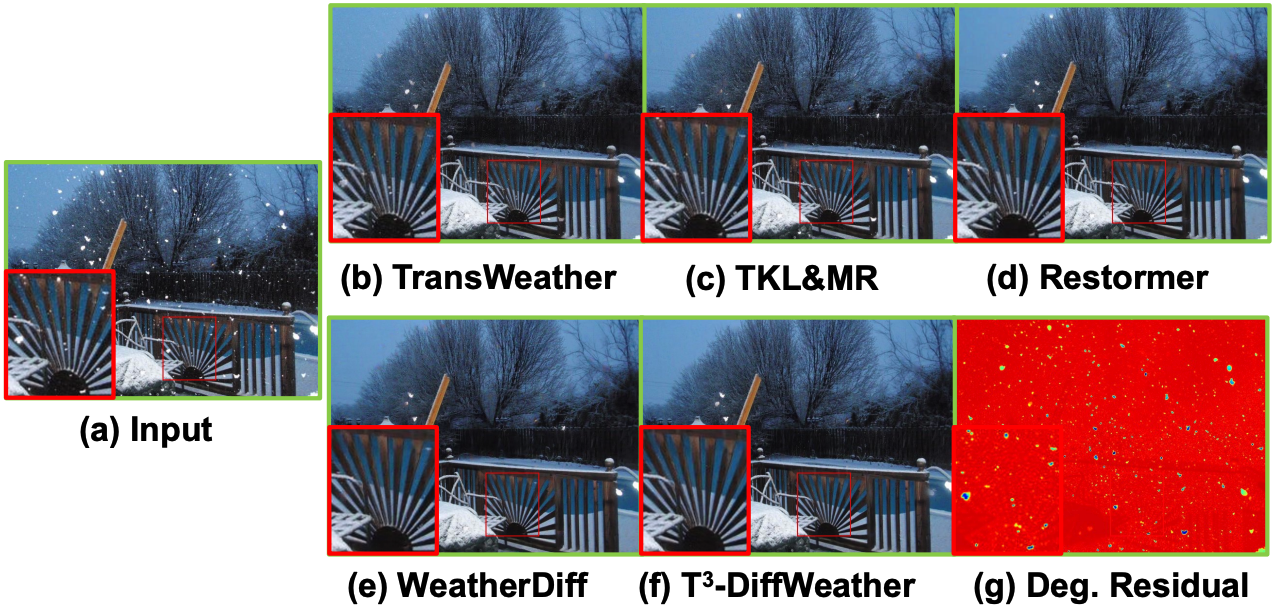
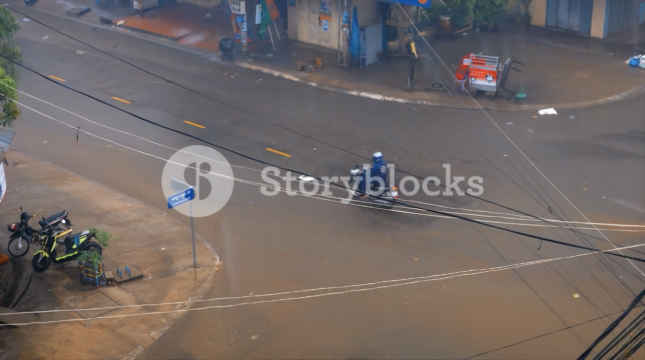
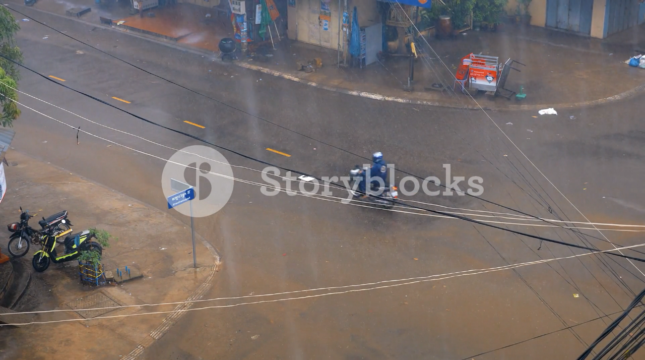
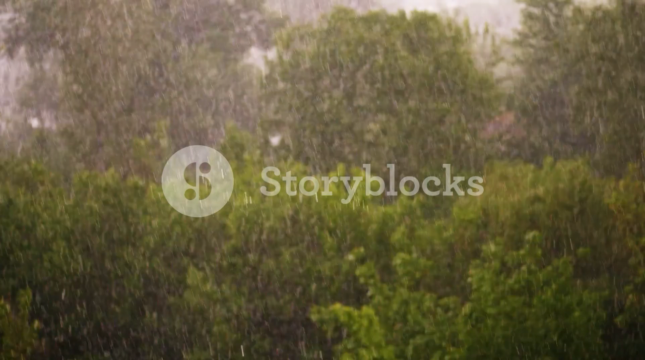
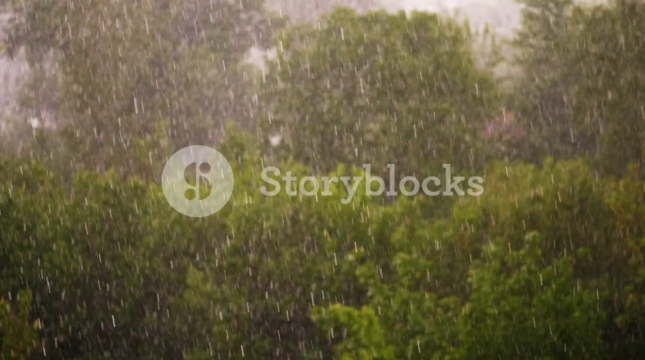
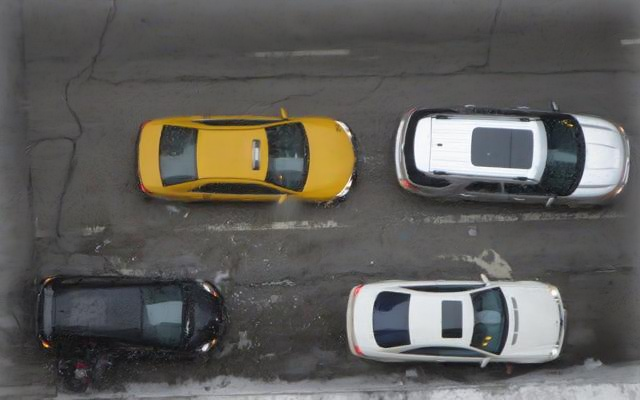
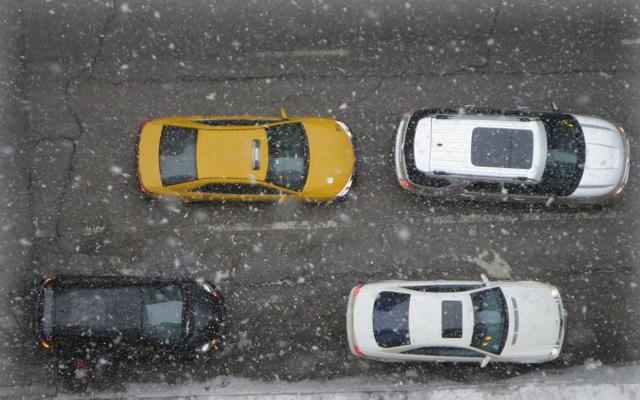
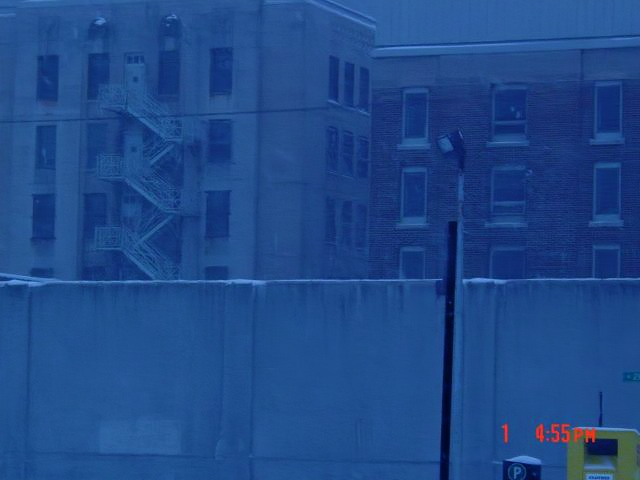
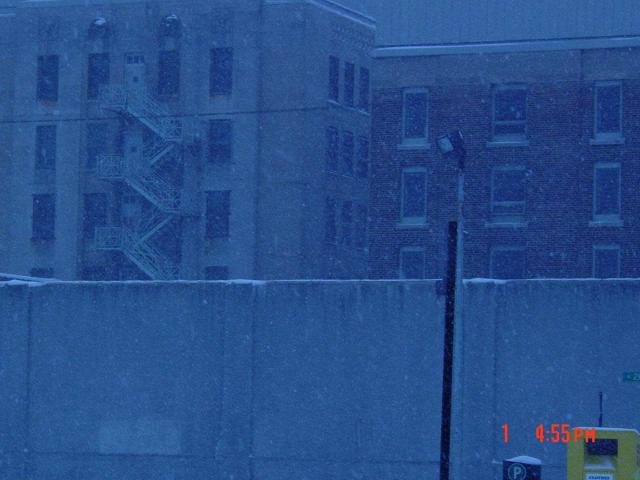
Citation
@InProceedings{chen2024teaching, title = {Teaching Tailored to Talent: Adverse Weather Restoration via Prompt Pool and Depth-Anything Constraint}, author = {Chen, Sixiang and Ye, Tian and Zhang, Kai and Xing, Zhaohu and Lin, Yunlong and Zhu, Lei}, booktitle = {European conference on computer vision}, year = {2024}, organization={Springer} }